A new automatic sentiment detection model for mentions: How does it work?
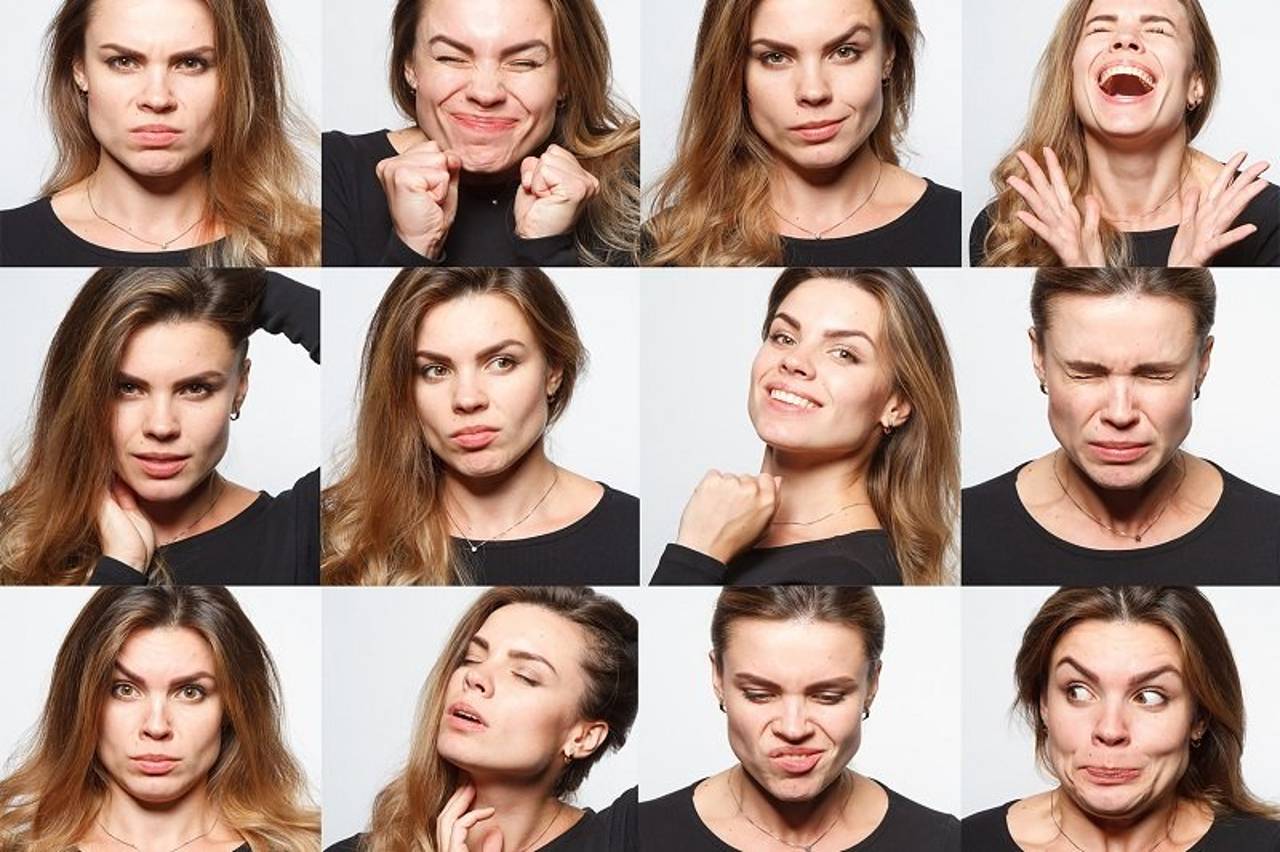
Recently prepared the most massive and – we're bold enough to say – revolutionary upgrade in YouScan's history for automatic sentiment detection.
Sentiment is one of the fundamental metrics of social media monitoring, and detecting it automatically can help you analyze the online landscape quickly and effectively. The automatic sentiment detection function has been part of YouScan's suite of services for a while, but we recently prepared the most massive and – we're bold enough to say – revolutionary upgrade in YouScan's history.
The new sentiment detection model is object-oriented, as before; but it also utilizes the latest deep neural network technology.
What does an "object-oriented model" mean?
A single post can express opinions about several objects in different contexts. The sentiment detection algorithm automatically identifies the subject of monitoring in the post, and determines the relevant sentiment specifically from the fragment of text pertaining the subject. When it comes to competitor analysis, the sentiment analysis will also vary depending on the brand that's being monitored.
90 - 95% accuracy
To train the new model, we used a selection of over 2 million processed posts from different fields, such as: taxi services, online stores, banks, medications, news, politics, sports, restaurants, beverages, mass consumer products, etc.
Currently, the detection accuracy of positive and negative mentions starts at 90%, and for thoroughly marked-up topics that contained a large number of mentions with clear sentiment, that number increases to 95% - which is a state-of-the-art indicator for this kind of task.
How can this model understand the context of the mention?
Another challenge of sentiment detection is the fact that the same word can carry both a negative and a positive meaning, depending on the context. For example, "Fairy is great for washing dirty pots" is a positive mention, while "Coca-Cola is great for washing dirty dishes" is a negative evaluation, since Coca-Cola isn't interested in being associated with a household cleaning product.
Our new sentiment detection model is great at addressing these challenges. There are deep neural networks at its basis, which have recurring memory layers, as well as layers that choose which part of the post need to be analyzed based on the context, subject and topic. Thanks to a large training data set, we were able to teach this model to understand the specifics of social media communication in order to determine sentiment for different brands according to their unique demands.
Does the model learn over time?
Will the quality of sentiment detection improve in certain monitoring topics if the sentiment in those topics is determined manually? Yes; we will update the model once a month, in order to re-train the algorithm with new data to understand new trends and keywords/phrases. So even when it comes to challenging new fields, we can make significant progress in improving the quality of sentiment detection.
Many YouScan users have already had a chance to get familiar with this new algorithm during beta-testing, and we've received extremely positive feedback. Starting today, the algorithm will be enabled for all accounts; please note that this might cause a spike in your quantitative indicators. The previous model was quite "conservative", and determined positive or negative sentiment only in cases of a high level of confidence or the presence of specific negative keywords. The new model offers a much more holistic approach to sentiment detection, so you may notice an increase in both positive and negative sentiment in topics with automatic sentiment detection. Please consider this in your data analysis.
We're always happy to hear your feedback about the new model. If you're not a YouScan user yet, request a demo today - and you too can test out the ability to automatically detect positive and negative sentiment in mentions of your brand.